How AI and Data are Shaping the Future of Scouting
—From Grassroots to Elite Clubs
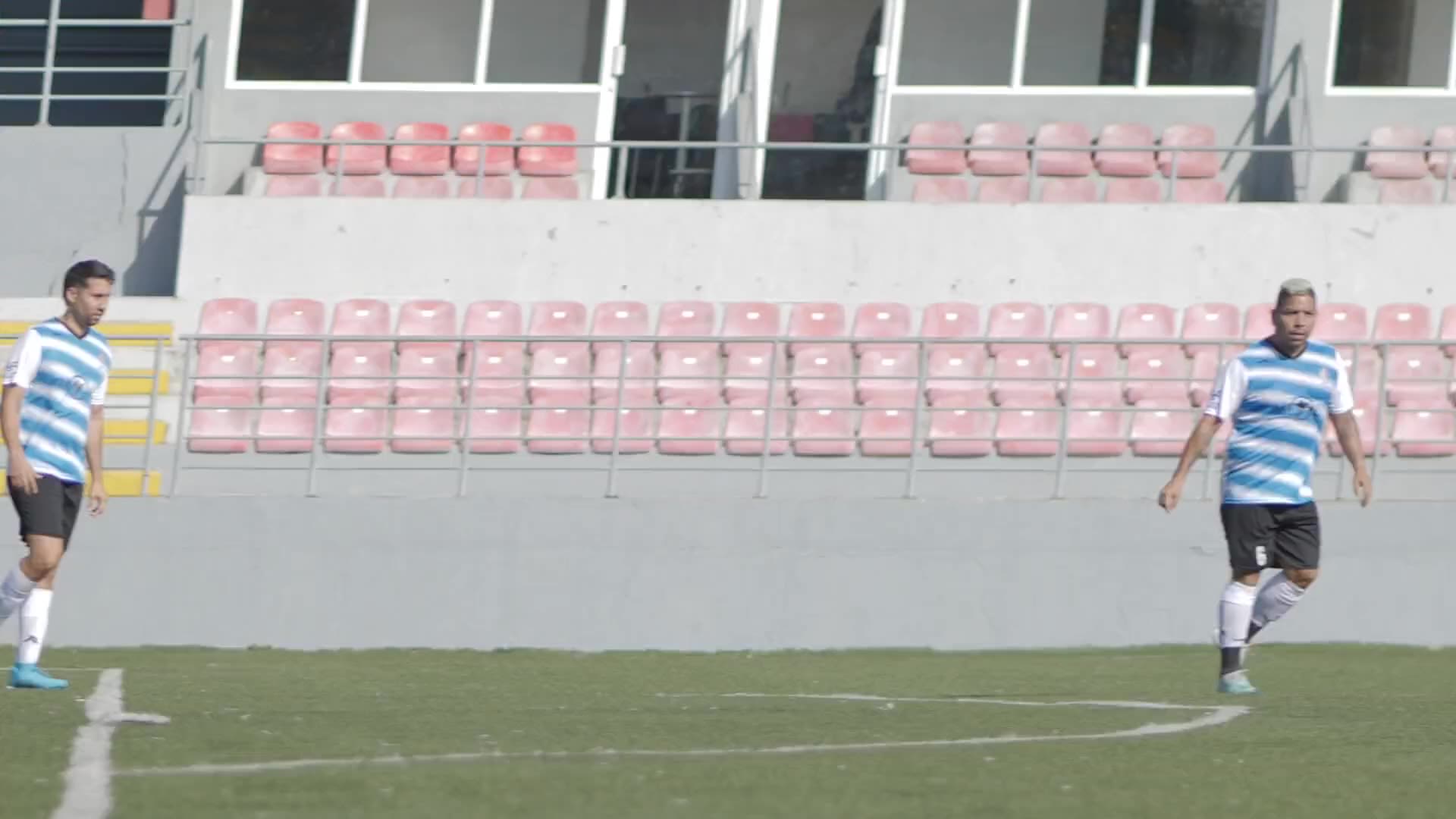
Introduction: From Instinct to Insight—The Data Revolution
in Football Scouting
In recent years, football scouting has transformed from an instinct-driven practice to a data-backed science. For clubs worldwide, leveraging data and artificial intelligence (AI) has enabled a shift toward strategic, objective recruitment. While powerhouse clubs like Manchester City and Bayern Munich rely on advanced scouting networks and data analytics, clubs with smaller budgets or limited resources are also harnessing these tools to uncover talent in underrepresented regions or lesser-known leagues.
The Foundation:
What is Data in Football?
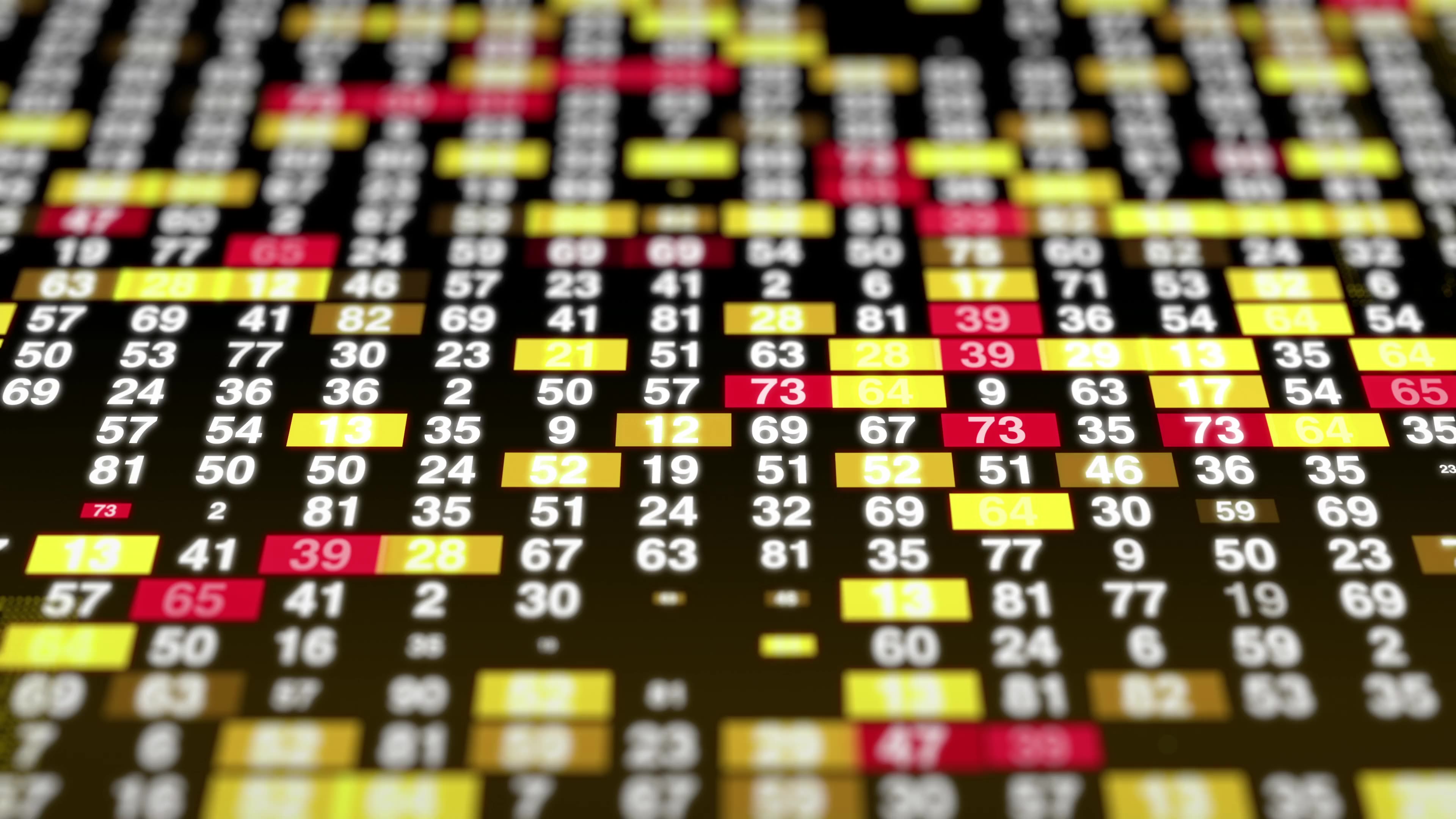
The term "data" refers to raw facts, figures, or information that is collected, observed, or measured from various sources. Data represents the qualitative or quantitative details of an object, event, or phenomenon, which can be organized, processed, and analyzed to draw conclusions, make decisions, or create insights. In a technological and scientific context, data is often stored digitally and manipulated using statistical, analytical, or computational techniques to transform it into meaningful knowledge.
In football, data is instrumental in enhancing both individual and team performance, collected from various sources both on and off the field. It encompasses metrics from players' actions during games—like goals, assists, or more complex metrics like Expected Goals (xG), and defensive recoveries—as well as data gathered through training sessions, physical assessments, and even wearable technology. This comprehensive data pool provides coaches, analysts, and players with valuable insights to inform training, optimize tactics, and support strategic decisions, all aimed at driving competitive success.
Studies in Journal of Sports Sciences explain that data can reveal intricate aspects of player performance, such as decision-making under pressure, consistency in passing accuracy, and even resilience in high-stakes moments.
There are three primary categories of data in football scouting:
Physical Data: Metrics on stamina, sprint frequency, and distance covered.
Technical Data: Measures like passing accuracy, shooting success, and pressing actions.
Tactical Data: Insights on off-ball movement, positioning, and reaction times, critical for assessing tactical fit.
However, managing vast amounts of data can be overwhelming and challenging to interpret effectively. This is where machine learning comes into play—by identifying patterns and making sense of complex data sets, machine learning helps streamline analysis, uncover hidden insights, and even make predictive recommendations. By leveraging these advanced tools, teams and analysts can transform overwhelming data into practical, actionable strategies, maximizing the potential of the information available.
Elite clubs use machine learning to process data and form predictive insights, as evidenced by studies from Carnegie Mellon’s AI Lab, which for example detail the use of algorithms to forecast players' success and injury risks.
Turning Data into Insight:
The Real Power of Data in Football
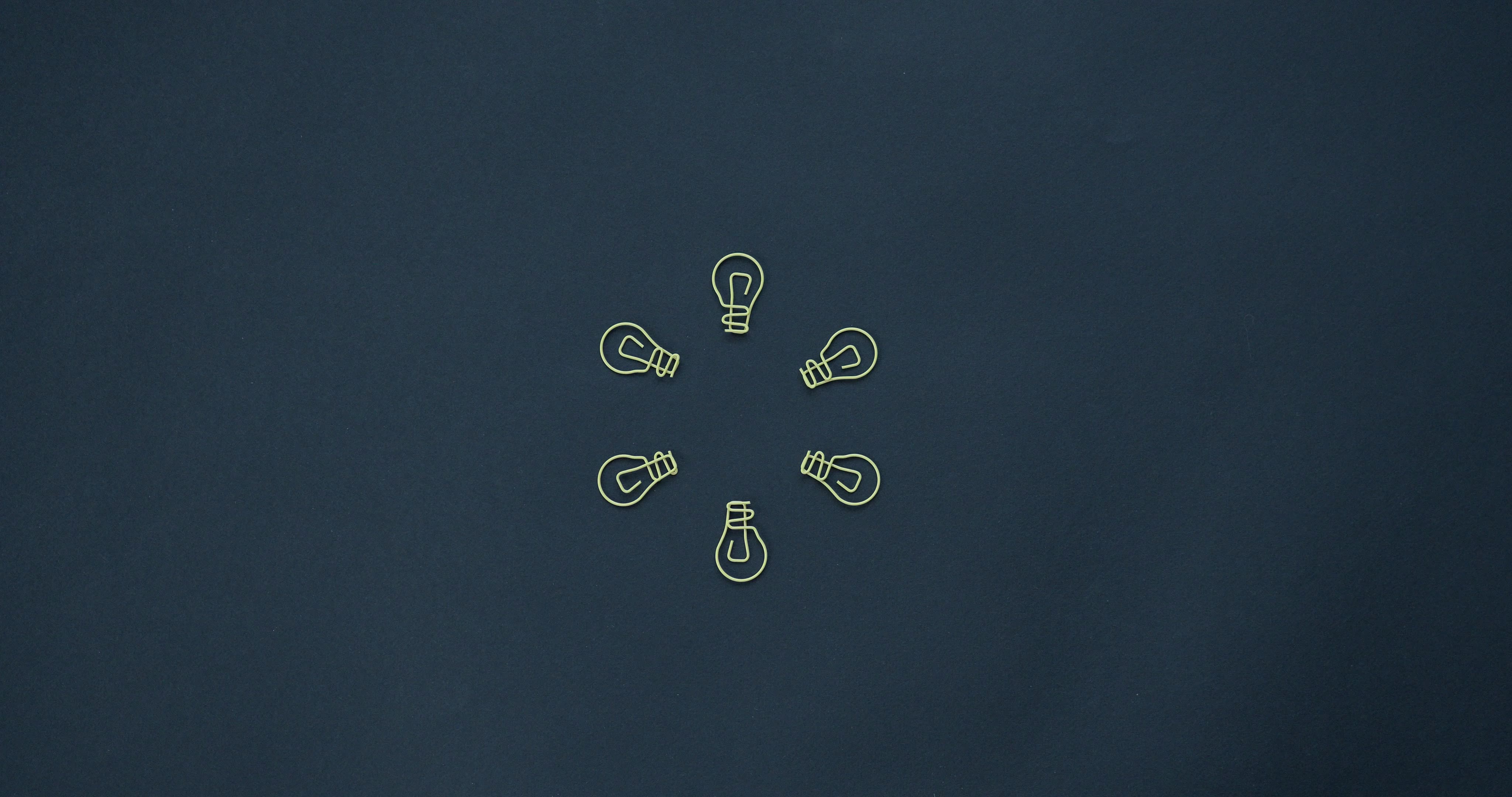
It's not about the data, its about the insight we can get from data. Converting raw data into actionable insight is at the core of effective scouting. Clubs increasingly focus on synthesizing data into clear, actionable insights—whether to predict a player’s tactical compatibility, growth potential, or resilience in critical moments.
At SL Benfica, a club known for its high-profile player development, scouts prioritize metrics that align with both technical excellence and adaptability, which are essential for players destined for international transfers. This approach has been widely covered in studies from the Portuguese Journal of Sports Science, highlighting the club’s method of blending data with qualitative assessments to maximize transfer value.
Integrating multiple data types like physical endurance, top speed, decision-making speed, and passing accuracy provides scouts with a holistic view of a player’s potential. Combining these metrics with video analysis offers a rich perspective on how a player might perform under specific tactical conditions.
At the end of the day, data serves as a powerful filter to identify potential transfer targets, helping clubs make informed decisions. It not only highlights promising players but also minimizes risks throughout the entire recruitment process by providing objective insights that complement traditional scouting. This strategic use of data supports clubs in making precise, data-driven investments aligned with team needs and goals.
Scouting Models Across Club Tiers:
From Global Giants to Grassroots
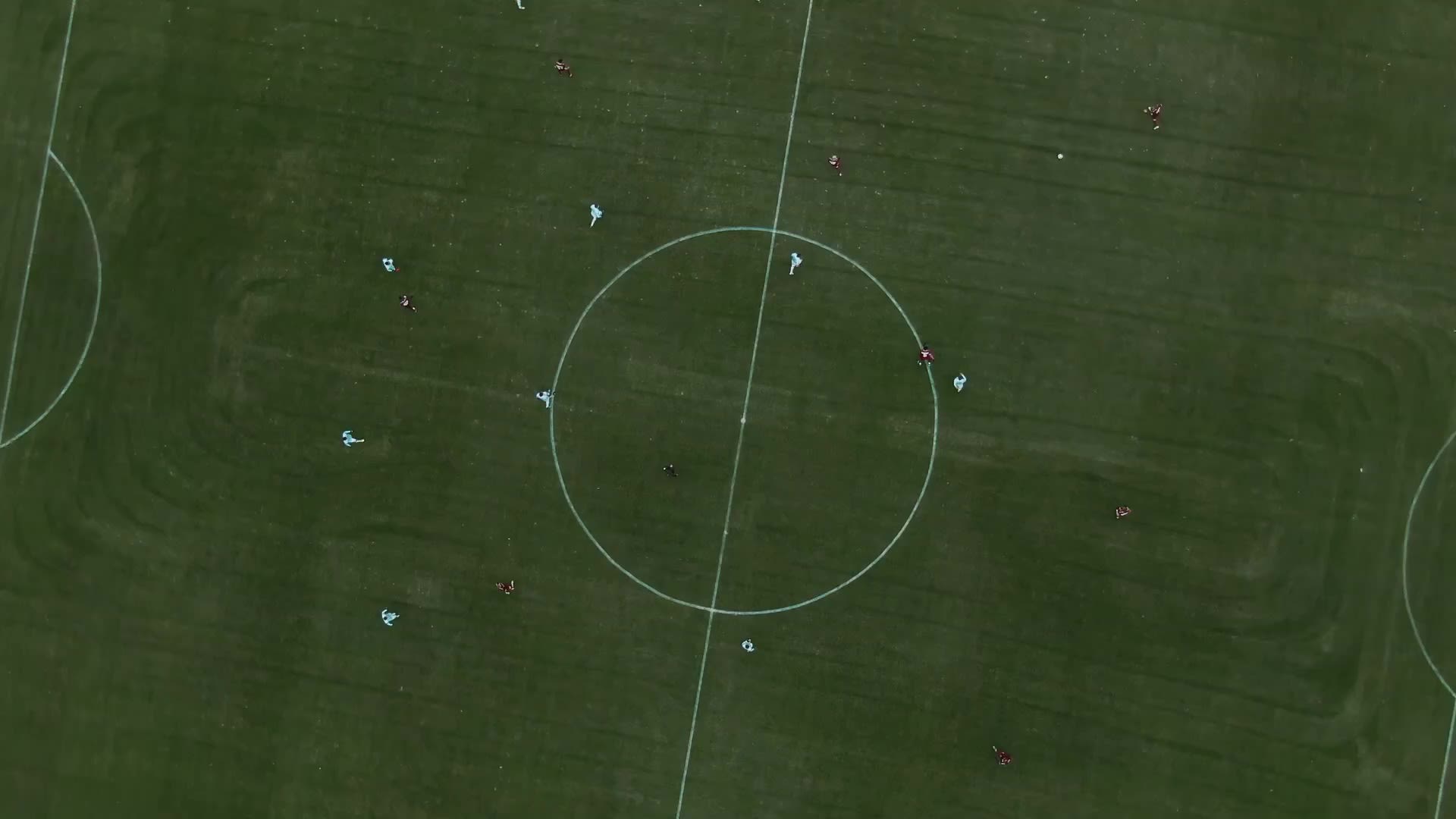
Elite Clubs: Sophisticated, Multi-Layered Scouting
Top-tier clubs like Manchester City, Liverpool, and FC Barcelona operate complex scouting systems. These clubs combine extensive data analysis with psychometric assessments, machine learning, and global scouting networks. For instance, Liverpool FC’s use of spatial analysis to measure player adaptability within their pressing system allows them to recruit players suited to specific tactical demands.
Scouting isn’t just about identifying talented players; it’s fundamentally about finding players who align with your playing style, team culture, and long-term vision. The ideal recruit not only brings skill but also enhances the team’s identity and cohesiveness, ensuring a seamless fit both on and off the field.
Mid-Tier Clubs with Data-Centric Approaches: Brentford and Midtjylland
Clubs like Brentford FC and FC Midtjylland have established themselves as industry leaders in data-driven recruitment. Brentford uses Expected Goals (xG) and pressing efficiency as core metrics, focusing on undervalued players who are often overlooked by traditional scouting methods. Studies in European Sports Management Quarterly have shown how this data-centric approach allows mid-tier clubs to identify high-potential recruits without overspending on star players.
Midtjylland, on the other hand, has pioneered the use of set-piece data to optimize scoring opportunities from corners and free kicks. Their approach has been shown to deliver consistent results in tight matches by maximizing high-probability scoring scenarios, as discussed in Football Data Review.
Lower-Budget Clubs and the One-Person Scouting Approach
In lower-budget leagues, such as Norway’s second tier, clubs often rely on network-based scouting, either due to limited resources or a lower prioritization of scouting infrastructure. Player evaluations are frequently managed by the sports director, a single scout, or an assistant coach, who rely heavily on personal contacts and regional networks to identify potential talent.
This approach, however, is not always effective; it can lead to missed opportunities, inefficiencies, and a heavy reliance on a limited network of contacts. While these networks remain invaluable, especially in leagues without the budgets for large investments, the smart application of AI can drastically optimize the scouting process. AI-driven analysis expands the pool of potential recruits, reduces dependency on specific contacts, and enables clubs to uncover talent that fits their needs more precisely—all while staying within budget constraints.
AI in Football Scouting:
Current Applications and Future Potential
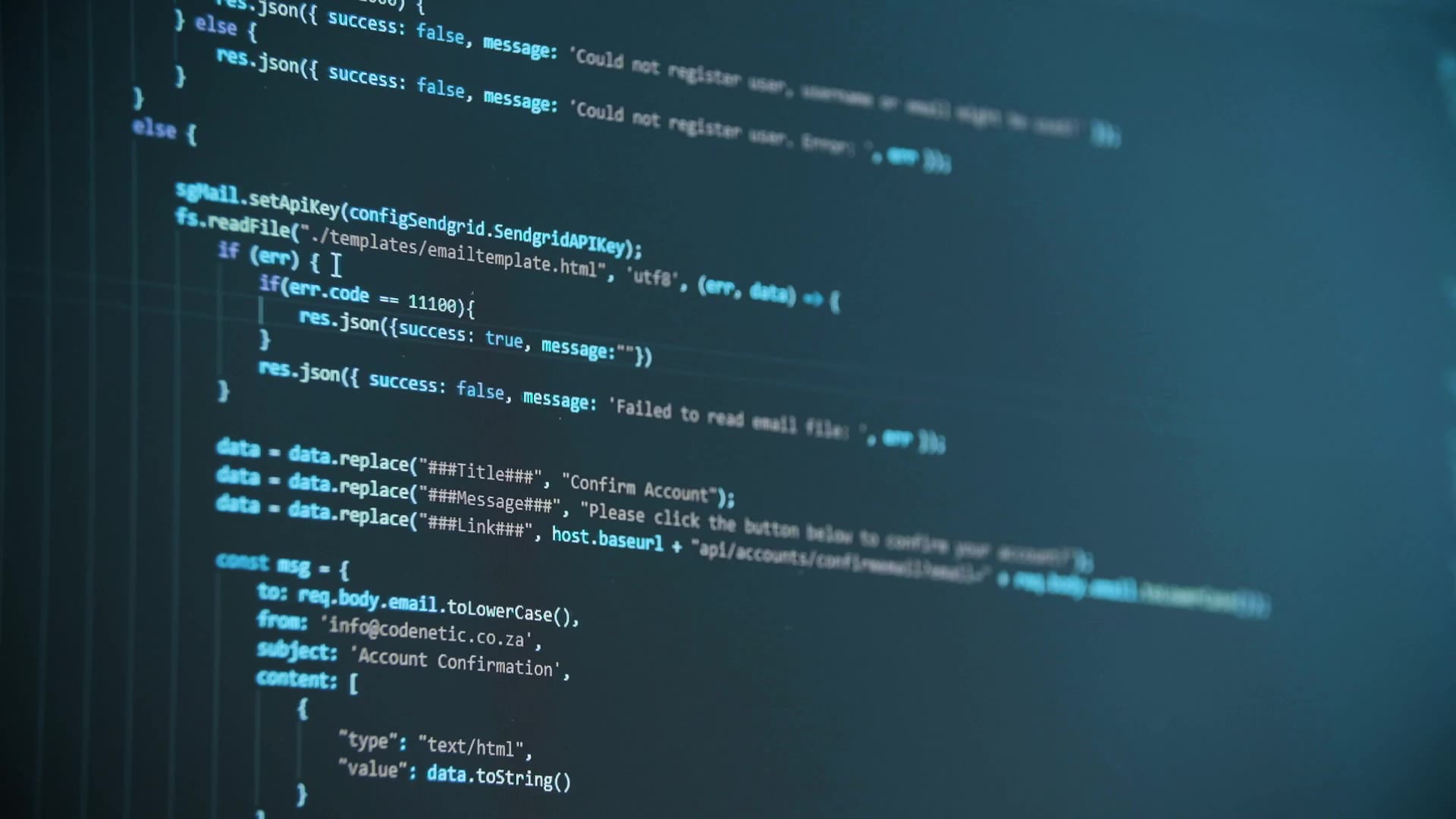
Artificial intelligence (AI) has become essential in football scouting, allowing clubs to turn raw data into actionable insights and efficiently analyze player performance and potential. By automating data processing and uncovering patterns, AI helps clubs make more informed recruitment decisions, optimizing the scouting process at every stage.
There are already numerous AI-driven tools readily available, making it easier than ever for clubs to integrate advanced data analysis into their scouting processes. These tools are capable of providing deep insights, from performance metrics to predictive analytics, allowing even resource-limited clubs to leverage sophisticated technology in player recruitment and development:
1. AI-Enhanced Video Analysis
AI-driven video platforms like Hudl and StatsBomb automatically tag player actions, allowing clubs to quickly analyze technical skills and tactical awareness. Clubs such as Arsenal and Chelsea have reported substantial time savings by integrating AI for action tagging and movement analysis.
2. Predictive Models for Career and Injury Trajectories
Machine learning models can predict career trajectories and injury risk based on historical performance and physical metrics. Manchester City, for example, uses AI to assess players’ physical endurance and recovery speeds, which inform scouting decisions focused on player durability.
3. Spatial and Tactical Awareness Evaluation
AI enables spatial analysis by identifying off-ball positioning and defensive structure. For instance, Liverpool uses AI to evaluate “football IQ” characteristics like positioning and reaction time, ensuring that recruits can integrate into their pressing-intensive system.
4. Psychological Profiling for Resilience and Adaptability
AI-driven psychometric assessments allow clubs to evaluate a player’s mental resilience, critical for high-stakes leagues. Studies in Journal of Applied Sport Psychology detail Borussia Dortmund’s use of these models to predict young players’ success in the Bundesliga, reducing risks associated with high-profile transfers.
The Future of AI in Scouting: What Lies Ahead?
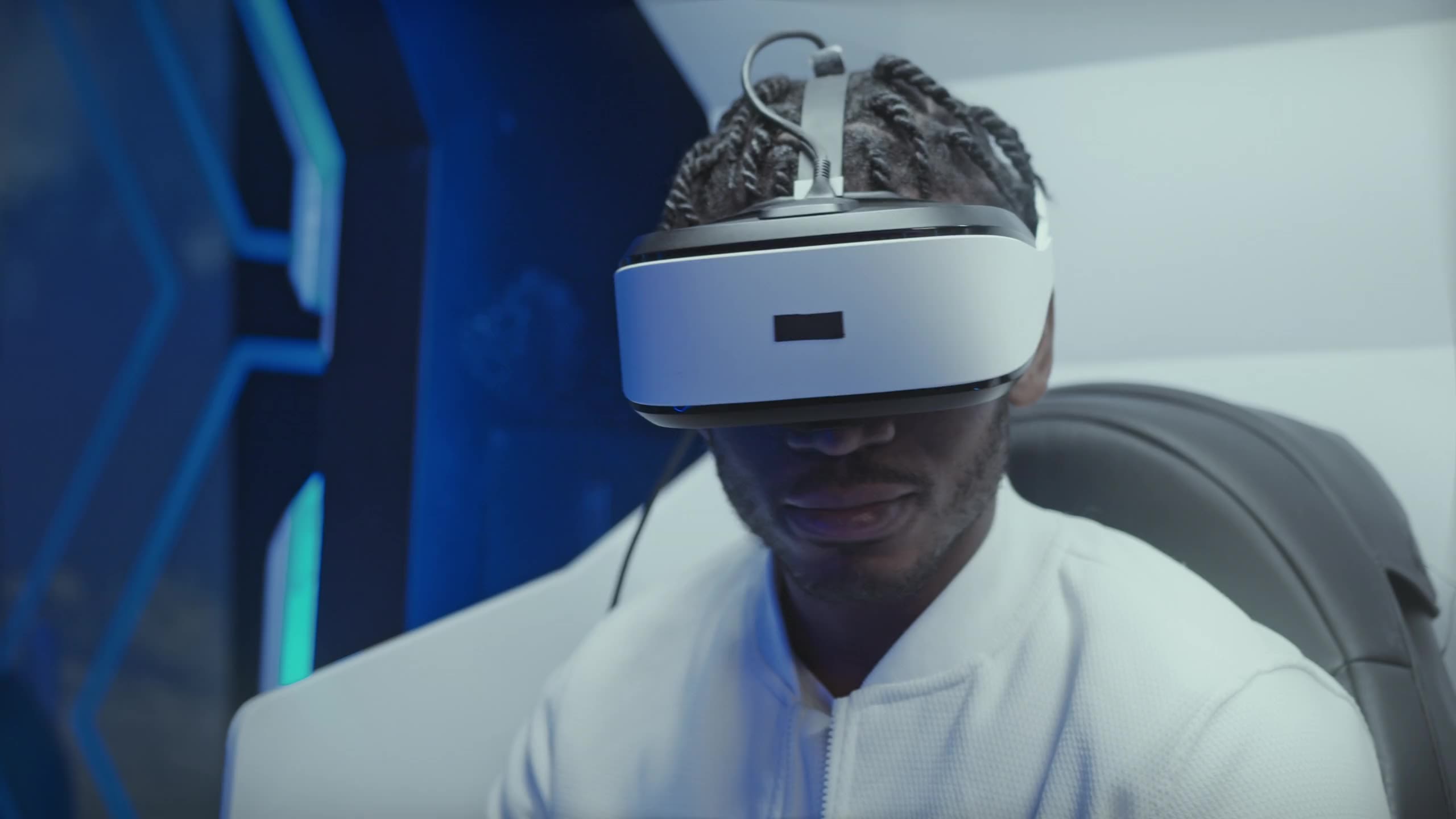
It's hard to know exactly what the future holds, but with AI, we can try to better predict developments with greater accuracy and confidence. To explore what's on the horizon, I asked ChatGPT about the possibilities ahead of us. Here are some of the ideas that arise - by the way, helping writing Scouting Reports are not on the list because that is something that if you are not doing already, then you don't know what you are missing!
- Analyze Real-Time Performance: Real-time AI analysis could assess players’ fatigue and tactical fit during games, suggesting in-the-moment adjustments for optimal performance and better player evaluation.
- Conduct Emotion Recognition for Pressure Situations: AI could monitor players’ emotional responses in high-stakes moments, providing insights into their mental resilience, which is critical in clutch situations.
- Simulate Virtual Reality Trials: VR technology combined with AI could enable clubs to hold remote trials, simulating real-game situations to assess decisional skill levels without geographical constraints.
- Predict Positional Shifts: AI could recommend future roles for young players based on their skills and physical attributes, allowing for early specialization or versatility planning. A player who plays in one position, with AI-driven analysis, can reveal hidden strengths and positional possibilities by examining performance metrics in-depth, enabling coaches to optimize a player's role on the team.
- Assist in Player Valuation and Negotiation: AI can provide clubs with accurate, data-driven insights into a player's market value, updating valuations based on recent performance, age, and comparable market trends. By tracking and analyzing metrics like consistency, impact in key moments, and long-term potential, AI assists in determining a player’s worth and helps clubs negotiate more effectively. These insights allow clubs to make strategic financial decisions, ensuring they neither undervalue their assets nor overpay for talent, ultimately optimizing budget allocation and roster composition.
Leveraging ChatGPT and Language Models in Scouting
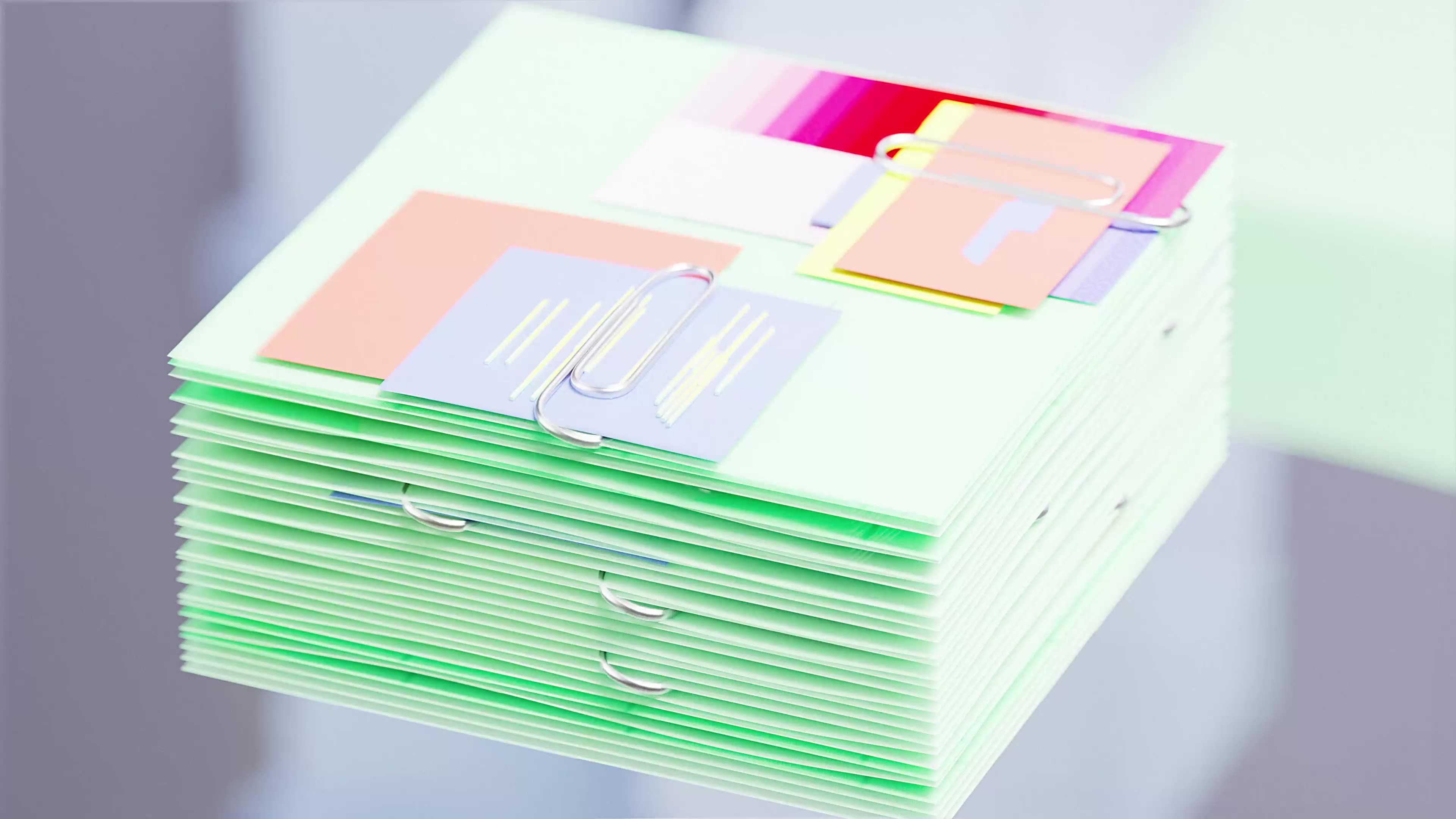
For clubs with limited resources, tools like ChatGPT are invaluable, making data-driven scouting accessible and efficient. By streamlining the tasks typically handled by a scouting department—such as player evaluation, travel logistics, budget allocation, report generation, and even communication—ChatGPT allows clubs to operate more strategically and cost-effectively. With these capabilities, OpenAI’s ChatGPT empowers clubs to:
- Summarize Reports Efficiently: ChatGPT can distill extensive scouting reports into key insights, saving time for decision-makers.
- Compare Players by Role: By inputting specific player data, ChatGPT can highlight differences and recommend which players may fit a given role better.
- Analyze Personality for Cultural Fit: ChatGPT can assess player personality traits from interviews or notes, helping clubs determine cultural compatibility.
- Organize Scouting Databases: Using ChatGPT to filter databases based on performance criteria simplifies the scouting process, allowing even one-person scouting teams to handle large datasets.
Example Prompt: "Compare Player A and Player B based on pressing actions, endurance, and goal-scoring ability. Which one fits better in a high-pressing forward role?"
And you can delve as deeply into specific details of 'what you’re after' as needed—provided you have datasets that can answer those questions. With the right data, your analysis can be as targeted and granular as the scouting objectives demand.
These applications allow clubs at all levels to access AI’s benefits without extensive infrastructure, making it easier to make data-driven, strategic recruitment decisions.
Conclusion: The AI-Driven Future of Football Scouting
AI and data have redefined football scouting, from elite clubs that employ multi-layered data and psychometric models to grassroots teams making the most of accessible tools like ChatGPT. As AI continues to advance, the potential for personalized, predictive scouting will expand, enabling clubs to build talent pipelines that align with both tactical and financial goals. The future of football scouting is smarter, faster, and more insightful than ever—revolutionizing how clubs uncover and develop football’s next generation of talent.
by HUGO VICENTE
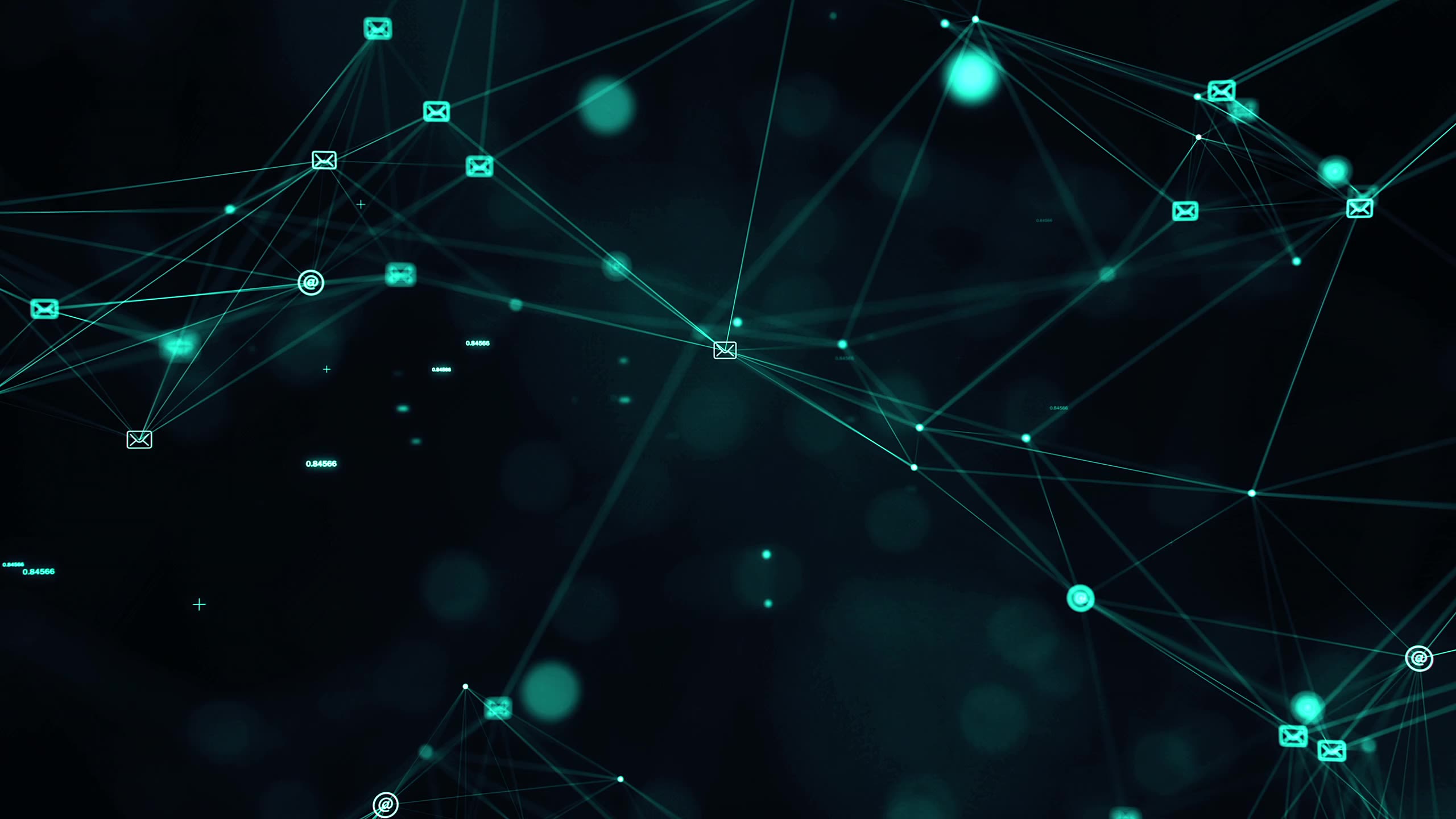
References
- A. B V, A. N. J, A. S. Gurikar, A. Joshi, A. Pandharkar and P. T R, "An xG Based Football Scouting System Using Machine Learning Techniques," 2024 IEEE 9th International Conference for Convergence in Technology (I2CT), Pune, India, 2024, pp. 1-3, doi: 10.1109/I2CT61223.2024.10544261.
- S. Venkataraman, K. Sundharakumar, A. Bharathi Malakreddy and S. Natarajan, "YUVA-SQ: A Cognitive Scouting Model for The Beautiful Game," 2024 5th International Conference on Innovative Trends in Information Technology (ICITIIT), Kottayam, India, 2024, pp. 1-6, doi: 10.1109/ICITIIT61487.2024.10580784.
- Malikov, D., & Kim, J. (2024). Beyond xG: A Dual Prediction Model for Analyzing Player Performance Through Expected and Actual Goals in European Soccer Leagues. Applied Sciences, 14(22), 10390. https://doi.org/10.3390/app142210390
- Mulazimoglu, O., Tokul, E., Can, S., & Eyuboglu, A. (2024). Examinando a superioridade dos times de futebol profissional com a contribuição do valor de gol esperado (xG). RBFF - Revista Brasileira De Futsal E Futebol, 16(64), 67-75. Recuperado de https://www.rbff.com.br/index.php/rbff/article/view/1391
- McCormack, S., Jones, B., Elliott, D., Rotheram, D., & Till, K. (2021). Coaches’ Assessment of Players Physical Performance: Subjective and Objective Measures are needed when Profiling Players. European Journal of Sport Science, 22(8), 1177–1187. https://doi.org/10.1080/17461391.2021.1956600
- Needham, C.J., Boyle, R.D. (2003). Performance Evaluation Metrics and Statistics for Positional Tracker Evaluation. In: Crowley, J.L., Piater, J.H., Vincze, M., Paletta, L. (eds) Computer Vision Systems. ICVS 2003. Lecture Notes in Computer Science, vol 2626. Springer, Berlin, Heidelberg. https://doi.org/10.1007/3-540-36592-3_27
- Aditya, R. S., & Yunus, M. (2024). Talent scouting and standardizing fitness data in football club: Systematic review. Retos: Nuevas Tendencias En Educación Física, Deporte Y Recreación, (60), 1382-1389.
- Pretto, F. (2022). Development of a football analytics web application for player scouting [Master's thesis, Universidad Torcuato Di Tella]. Universidad Torcuato Di Tella Repository. https://repositorio.utdt.edu/handle/20.500.13098/11892
- Radicchi, Elena & Michele, Mozzachiodi. (2016). Social Talent Scouting: A New Opportunity for the Identification of Football Players?. Physical Culture and Sport : Studies and Research. 70. 10.1515/pcssr-2016-0012.
- Woods, C. T., Joyce, C., & Robertson, S. (2016). What are talent scouts actually identifying? Investigating the physical and technical skill match activity profiles of drafted and non-drafted U18 Australian footballers. Journal of Science and Medicine in Sport, 19(5), 419-423. https://doi.org/10.1016/j.jsams.2015.04.013
- Memmert, D., & Raabe, D. (2018). Data Analytics in Football: Positional Data Collection, Modelling and Analysis (1st ed.). Routledge. https://doi.org/10.4324/9781351210164
- Guimarães, J. H. M. M. (2018). Data analytics applied to football and football players [Master's thesis, Universidade Católica Portuguesa]. Veritati - Repositório Institucional da Universidade Católica Portuguesa. http://hdl.handle.net/10400.14/27626 1
- https://www.ml.cmu.edu/research/
- Pavitt, Joe & Braines, Dave & Tomsett, Richard. (2021). Cognitive Analysis in Sports: Supporting Match Analysis and Scouting through Artificial Intelligence. Applied AI Letters. 2. 10.1002/ail2.21.
- Efe, Doç. Dr. Ahmet. (2023). An Assessment Over the Impact of Artificial Intelligence on Sports Activities and the Sports Industry.
- Z. Pu, Y. Pan, S. Wang, B. Liu, M. Chen, H. Ma, and Y. Cui, “Orientation and decision-making for soccer based on sports analytics and AI: A systematic review,” IEEE/CAA J. Autom. Sinica, vol. 11, no. 1, pp. 37–57, Jan. 2024. doi: 10.1109/JAS.2023.123807
- Wang, Z., & Veličković, P. (2024, March 19). TacticAI: An AI assistant for football tactics. DeepMind. https://deepmind.google/discover/blog/tacticai-ai-assistant-for-football-tactics/
- D. Patnaik, H. Praharaj, K. Prakash and K. Samdani, "A study of Prediction models for football player valuations by quantifying statistical and economic attributes for the global transfer market," 2019 IEEE International Conference on System, Computation, Automation and Networking (ICSCAN), Pondicherry, India, 2019, pp. 1-7, doi: 10.1109/ICSCAN.2019.8878843.
- M. A. Al-Asadi and S. Tasdemır, "Predict the Value of Football Players Using FIFA Video Game Data and Machine Learning Techniques," in IEEE Access, vol. 10, pp. 22631-22645, 2022, doi: 10.1109/ACCESS.2022.3154767.
- Behravan, I., Razavi, S.M. A novel machine learning method for estimating football players’ value in the transfer market. Soft Comput 25, 2499–2511 (2021). https://doi.org/10.1007/s00500-020-05319-3
- E. Choustoulakis, E. Pastelakos (2024) AI-DRIVEN ANALYSIS OF GAME TACTICS AND PLAYER PERFORMANCE, ICERI2024 Proceedings, pp. 3350-3359.
- Benito-Santos, A., Theron, R., Losada, A., Sampaio, J. E., & Lago-Peñas, C. (2018). Data-driven visual performance analysis in soccer: An exploratory prototype. Frontiers in Psychology, 9, 2416. https://doi.org/10.3389/fpsyg.2018.02416
- Goes, F. R., Meerhoff, L. A., Bueno, M. J. O., Rodrigues, D. M., Moura, F. A., Brink, M. S., … Lemmink, K. A. P. M. (2020). Unlocking the potential of big data to support tactical performance analysis in professional soccer: A systematic review. European Journal of Sport Science, 21(4), 481–496. https://doi.org/10.1080/17461391.2020.1747552
- Link, D. (2018). Data analytics in professional soccer: Performance analysis based on spatiotemporal tracking data. Springer Vieweg Wiesbaden. https://doi.org/10.1007/978-3-658-21177-6